Filter bubbles, bias, rabbit holes and nudging
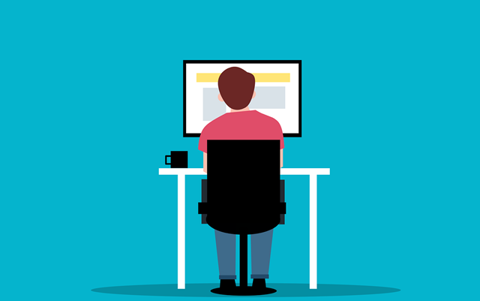
About this lesson
This lesson focuses on the AI systems that recommend content in various applications that students use on a day-to-day basis. It draws on students’ ethical understandings during analysis of these systems. This lesson was developed by the Digital Technologies Institute in collaboration with the Digital Technologies Hub.
Year band: 9-10
Curriculum Links AssessmentCurriculum Links
Links with the Digital Technologies Curriculum Area.
Year | Content Description |
---|---|
9-10 |
Develop techniques to acquire, store and validate data from a range of sources using software, including spreadsheets and databases (AC9TDI10P01) Apply the Australian Privacy Principles to critique and manage the digital footprint that existing systems and student solutions collect (AC9TDI10P14) Analyse and visualise data interactively using a range of software, including spreadsheets and databases, to draw conclusions and make predictions by identifying trends and outliers (AC9TDI10P02) Evaluate existing and student solutions against the design criteria, user stories, possible future impact and opportunities for enterprise (AC9TDI10P10) |
Relevant Achievement Standard
They evaluate information systems and their solutions in terms of risk, sustainability and potential for innovation and enterprise. |
Assessment
Teacher assessment
Task 1:
Provide a set of questions to pose before and after studying AI recommender systems.
Some examples include:
- What is innovative about AI recommender systems, and how do they work?
- How might you need to protect your privacy and personal information when interacting with an AI-powered recommender system?
- What data does an AI recommender system use?
- What are some potential issues of an AI recommender system?
- How would you model or visually represent how an AI works – for example one that suggests movies to view?
Task 2:
Present a comparison on two different users of an online system that uses an AI recommender to suggest content that the use is likely to be interested in. The comparison can include a mockup of a screen for each user displaying the relevant online content. Explain how the system is innovation and also identify any risks.
Use each student’s artefacts developed through the study to assist in assessing their knowledge, skills and processes.
Guide to assessment
Achievement standard | Assess student response to these questions | Assess student artefacts |
---|---|---|
They evaluate information systems and their solutions in terms of risk, sustainability and potential for innovation and enterprise. |
||
What is innovative about an AI recommender system and how do they work? |
Student response to Task 2 Student completed worksheet: Recommender systems |
|
How might you need to protect your privacy and personal information when interacting with an AI powered recommender system? |
|
|
What are some potential issues of an AI recommender system? |
Student presentation of their independent research and slide deck |
|
Content descriptions |
||
Develop techniques for acquiring, storing and validating quantitative and qualitative data from a range of sources, considering privacy and security requirements |
What data does a recommender system use? |
Interaction with movie database |
Analyse and visualise data to create information and address complex problems, and model processes, entities and their relationships using structured data |
How would you model or visually represent how an AI works; for example, one that suggests movies to view? |
Student completed Worksheet: AI movie recommender system |
Preparation
Key terms
Artificial intelligence (AI) | The ability of machines to mimic human capabilities in a way that we would consider 'smart'. |
---|---|
Machine Learning | An application of AI. A process in which we feed the machine many examples of data that demonstrate what we would like it to do, so that the machine can itself work out how to achieve a goal. The machine learns and adapts its strategy to achieve this goal. |
Recommnder system | Recommender system or a recommendation system is a type of information filtering system that attempts to predict the rating or preference a user would give to an item. |
Ethical issue | A situation where there are competing alternatives and the right thing to do is not obvious or clear. Sometimes terms such as good, bad, wrong, better or worse are used to consider the effect of particular actions on our lives, society, nature and the environment. |
Ethical behaviour | Behaviour that fits accepted standards of conduct. |
Learning map and outcomes
Develop an understanding of the technical and economic interests behind recommender systems and the ethical and societal implications that they cause.
Adapt this learning map to suit your students’ needs.
By the end of this lesson students will: | Mindset | Skillset | Toolset |
---|---|---|---|
Develop an understanding of information systems that include recommender systems. |
Analytical, Reflective, Curious |
Collaborating, Clarifying |
Google Form or similar tool to collect data on student app use Worksheet 1: AI information systems |
Develop an understanding how an AI uses data to provide its recommendations |
Analytical, Reflective, Curious |
Collaborating, Clarifying |
Worksheet 2: AI movie recommender Database |
Explain how AI recommender systems can impact on society both positively and negatively. |
Analytical, Reflective, Curious |
Collaborating, Clarifying |
Student independent research and student-created slide deck |
Learning hook
Student app use
Use unidentifiable student-collected data to explore the impact that smartphones, tablets and other digital systems have on our daily lives. Many digital applications that we use incorporate an AI system.
As part of a flipped lesson, students gather data and bring it to class or complete an online form before the lesson.
Pose this question: What app is the most commonly used by students in our class, and on average how much time would be spent on this app per day?
Demonstrate that most smartphones display the time spent per day on various applications.
Suggest that students view their own data usage and share this with the class to contribute to a class display of that data using de-identified data.
Ask your students to share data anonymously via an online form such as Google Forms.
Students complete a survey question which might include the following (but remember that some may change in currency for this age group over time, and new ones may need to be added):
Survey question example
In the table below, insert the time in minutes that you would spend on an average weekday on particular mobile apps or the internet.
Applications | 0 min | Up to 30 min | Between 30 min and 60 min | More than 60 min |
---|---|---|---|---|
SMS Facebook Messenger Snapchat TikTok Internet search/browsing YouTube other |
Reference: What is Apple Screen Time and how does it work?
Use the think-pair-share strategy to list the reasons why students spend time on these things.
Use prompts such as:
- What is the purpose of the app or use of the internet?
- How might some applications or websites attract your attention?
- Why might some apps or websites want you to view their content or use their service?
Ask students to consider these terms:
- rabbit hole, filter bubble, nudging.
Have each group write a brief description of what they think each means in the context of browsing the internet, using social media and viewing online content.
Learning input
Introduce AI applications
Explore the way in which many of the systems we use on a daily basis incorporate an AI recommender system.
Introduce the following AI information systems. Discuss the services with which students are familiar. Discuss as a class or print the worksheet ‘Recommender systems’.
Information systems (incorporating a recommender system) | What did you notice about the content recommended to you by the system? | Why do you think that content in particular was suggested? | What strategies did the system use to attract you and keep you using their content or service? |
---|---|---|---|
Video streaming Video streaming services include: YouTube, Netflix, Disney+, Stan. |
|||
Social media Social media services include: Facebook, Instagram, Snapchat. |
|||
Online shopping Online shopping includes: eBay, Amazon, AliExpress. |
|||
News sites News sites include: Buzzfeed, Reddit or news via you might receive via social media. |
Questions
Ask students these questions.
- How do you think the system comes up with its suggestions?
- Did you think the suggestions were relevant for you? Why or why not?
- What might be a drawback of these particular systems?
According to a report, on digital use globally (Jan 2021) it is estimated that there are around 5 billion internet users. About 4 billion of them are active social media users. Every minute, 4.2 million search queries are entered into web browsers and 460,000 tweets are constructed. The amount of data that is created or shared on the internet exceeds a single person’s ability to absorb it all. This information overload necessitates strategies and tools to manage, organise, structure, and filter information based on the likely relevance for the user. In pre-internet times, newspapers performed a limited role of procuring, analysing, summarising and finally printing articles for their readers. Based on their interests, people would prefer one over the other newspaper. But if they could, many would try to read at least two newspapers, to get a balanced view of current affairs.
According to a Business Insider article Feb, 2018, Netflix is estimated to have 5,600 titles in its catalogue. Unless we knew in advance exactly which movie or TV series we wanted to watch, we would not be able to browse it within a reasonable amount of time to find a movie you’d like. Automatic recommender systems manage the enormous amount of data on video streaming services, social media platforms, or online shops and filter thousands if not millions of options to just a handful that we then decide on.
Learning construction 1
Explore a simple movie recommender system
Students explore the concept of recommender systems using a simple AI movie recommender system that contains information (metadata) related to six movies. Access this system at mycomputer brain.net.
Provide students with the worksheet ‘AI movie recommender system’. The worksheet guides their investigation of a simple AI. It covers:
- what they notice about the AI (which allows for student interest and catering for a range of views)
- applying their understandings of an AI to the concept of creating an account and determining preferences to ‘jump start’ the recommendations system
- Use a bubble map to represent data an AI might use to determine what titles you might like to view. How might this be connected to data from other members? Show these connections too!
Metadata about a movie is the information that describes a movie, such as its title, genre and a synopsis of the movie. For example, the movie Ratatouille (title) is an animated (genre) comedy (genre) family (genre) movie about a rat who can cook and who makes an unusual alliance with a young kitchen worker at a famous restaurant (synopsis).
Follow the instructions (Mission Briefing) on the right-hand side menu.
Screen capture from mycomputerbrain.net Machine learning: identifying movies with AI with mission briefing
Instead of writing a program that has many IF … THEN … ELSE statements, and just by giving this AI data samples, the AI has made the connection between genres and movie titles. This is a very flexible method that scales up well to deal with thousands of movies.
This sample AI is, of course, a very simple example. To determine which movies a user might like, commercial AI systems use information such as:
- age/viewing restrictions
- how the user rated other titles
- viewing history
- information related to each title, such as genre, categories, actors, year of release
- other members with similar preferences.
Learning construction 2
Explore potential issues of an AI recommender system
Provide students with the research task of exploring an AI recommender system’s potential problems. Students could research a topic of interest.
Ethical questions
An AI makes suggestions purely on the basis of its training and its input data. Here are some possible avenues of exploration.
- If an AI recommender system largely shows content that is similar to things that a person already likes, what could be the result?
- What are the possible implications for our society if everyone just sees the news that corresponds to their view of the world?
- Democratic systems require a respectful but frank and open debate about issues. For a balanced debate that considers many different aspects of an issue, it is important to consider different views. This is distinct from expressing the same issue in different ways. What might be the positive and negative implications of news recommender systems on democracy?
Potential issues: filter bubbles, bias, rabbit holes and nudging
Filter bubbles
- A filter bubble is a state of isolation that can result when a recommender system only provides the type of information that a user is already interested in. For example, only climate-change affirming news is presented to a climate activist or, conversely, only climate-change critical information to a climate-change sceptic. You can pick any topic of social relevance and imagine a black and white information pattern that is either exclusively positive or negative.
A filter bubble can lead to – often unconscious – bias when a person comes to think that their view of the world is balanced and therefore a true reflection of the world. The underlying fallacy (false or mistaken idea) of that person’s belief is that reading many different articles within their filter bubble (expression of the same thing in many different ways), is the same thing as exploring different views.
This bias is based on a filtered data set provided by the recommender system. It is important to understand that any data set is biased. This is because to make a subset of anything you have to apply selection criteria (filters). Once you filter something, you introduce bias. Filtering means removing. Imagine filtering ground coffee in a coffee machine. The filter will remove coarse-grained particles because we don’t like drinking them. Of course, we do this to enjoy coffee. So a filter has a purpose; we just need to be conscious that it is there.
Rabbit hole
- In social media, a rabbit hole is a situation in which we get dragged into a chain of related posts. This often starts when we look at a post and then see related posts presented by a recommender system. Our brain likes similar things, so we like to follow similar things. You have probably experienced this when you watched a chain of related funny videos on YouTube. We get quite time-blind when we do this and can spend a large amount of time going down the rabbit hole. The term ‘rabbit hole’ was first introduced in the book Alice’s Adventures in Wonderland by author Lewis Carroll.
Nudging
- Nudging is the technique of influencing the behaviour of a person in subtle ways. In a social media context, this is the placement of posts and advertising that resonates with the user. The nudge alters a person’s behaviour in a way towards a desired behaviour.
Observe how social media will try to nudge users into buying products, voting for parties, joining campaigns and social causes. A nudge may or may not be in the best interests of the nudgee. It is good practice to recognise nudging attempts and to evaluate them critically.
Students prepare a slide deck with four or five slides, which they use to present their research findings to the class or within small groups.
Learning construction 3
Dealing with data
Students can experience interrogating large amounts of data using spreadsheets or databases. These techniques are not AI-based, but convey the general understanding of processing data with algorithms. Students unfamiliar with databases can use the spreadsheet of the IMDB data and use filter and sort to refine search queries.
The following example uses a database developed using Microsoft Access.
For non-Windows users save the spreadsheet file and upload using your school’s designated database software.
Database approach
A cleaned version of a subset of the IMDB database, with inappropriate references removed. Download the database. Open the database with Microsoft Access (only available for Windows operating systems).
We can run queries in the Structured Query Language (SQL) to interrogate the data. Two queries have been included to enable students to explore the information. Select a query and double click to view the results returned. As students explore the database ask them what queries they could ask of the data.
Student suggestions for queries may include: | Suggested query | Tips |
---|---|---|
What are the most popular movies? |
SELECT [IMDB Movie Data].Movie_Name, [IMDB Movie Data].Genre, [IMDB Movie Data].Year, [IMDB Movie Data].Rating, [IMDB Movie Data].Metascore FROM [IMDB Movie Data] ORDER BY [IMDB Movie Data].Rating; |
‘Select’ indicates the headings you want to show in results. |
What are the most popular action and adventure movies? |
SELECT * FROM [IMDB Movie Data] WHERE (([IMDB Movie Data].Genre) Like "*Adventure*" AND ([IMDB Movie Data].Genre) Like "*Action*") ORDER BY [IMDB Movie Data].Metascore DESC; |
Here an (*) after ‘Select’ is used to show all headings in results. Syntax: use single or double quotes and an *to indicate search field item eg "*Adventure*" Note that the two genres – adventure, action– are combined with a logical AND operation. This means that only movies that contain a genre of both action and adventure are included in the result. ‘Metascore DESC’ means the results will be sorted highest to lowest. |
Are there any movies directed by James Cameron? |
SELECT Movie_Name, Genre, Director FROM [IMDB Movie Data] WHERE [IMDB Movie Data].Director Like '*James Cameron*'; |
|
I like all other genres but hate Sci-Fi. What popular movies would you recommend? |
SELECT [IMDB Movie Data].Movie_Name, [IMDB Movie Data].Genre, [IMDB Movie Data].Year, [IMDB Movie Data].Rating, [IMDB Movie Data].Metascore FROM [IMDB Movie Data] WHERE [IMDB Movie Data].Genre Not Like '*Sci-Fi*' ORDER BY [IMDB Movie Data].Metascore DESC; |
Use NOT operation to exclude results. |
Example of the IMDB database in Microsoft Access
Select ‘Query 1’, right click and select ‘Design view’
This displays the current query.
Type changes to the search parameters.
Be careful to keep the syntax (use of * and “ etc)
Select ‘Run’ to view query results.
Example of a query in design view in the IMDB database in Microsoft Access
Alternate view
The ‘Design view’ can also present the query to modify as a table.
In this view drop down menus enable the user to modify parameters.
Make changes to search queries.
Select run to show results.
Example of an alternate view of a query in design view in the IMDB database in Microsoft Access
Resources
- Digital 2021: Global overview report Stats about global digital usage
- Worksheet 1: Recommender systems
- Worksheet 2: AI movie recommender system
- IMDB database MS Access database file
- IMDB Excel data
- My computer brain AI tool for exploration based on movie selection
- Everything you always wanted to know… A lesson idea to enable students to learn how to create your own database